Vahid Reza Gharehbaghi: Pioneering Structural Health Monitoring in Civil Engineering
Revolutionizing Damage Detection Techniques for Modern Infrastructure
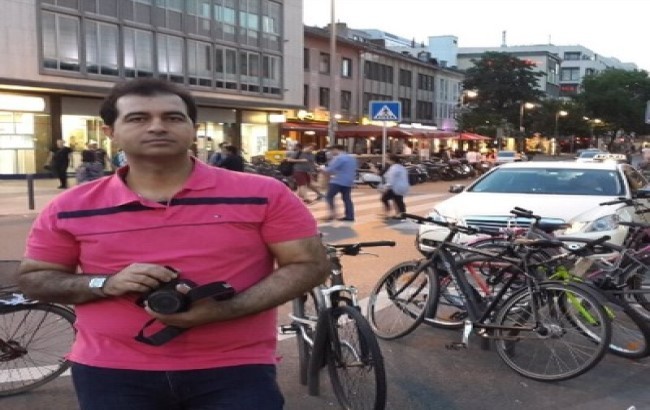
Vahid Reza Gharehbaghi is a distinguished civil engineering figure known for his groundbreaking contributions to structural health monitoring and damage detection in building structures. His innovative approaches, leveraging advanced machine learning and deep learning techniques, have significantly improved the accuracy and efficiency of identifying structural damages, ensuring the longevity and safety of modern infrastructures.
Introduction
Structural health monitoring (SHM) has become an essential component in maintaining and ensuring the safety of civil infrastructure. Technological advancements have enabled more sophisticated methods to detect and assess damages in structures, ensuring timely interventions and reducing risks. Among the pioneers in this field, Vahid Reza Gharehbaghi stands out for his innovative contributions, particularly in applying machine learning and deep learning techniques for structural health monitoring.
Background and Academic Contributions
Educational Background and Professional Affiliations
Vahid Reza Gharehbaghi is affiliated with the Department of Civil Engineering at the University of Kansas, United States. His academic journey and professional endeavours have been dedicated to advancing the field of structural health monitoring, focusing on integrating new technologies to enhance the detection and assessment processes.
Key Research Areas
- Damage Detection Using Machine LearningGharehbaghi’s research primarily revolves around using machine learning algorithms to identify and analyze structural damages. His notable work includes the application of variational mode decomposition (VMD) and the GARCH model to detect both linear and nonlinear damages in structures.
- Deep Learning in Structural Health Monitoring Another significant area of Gharehbaghi’s research is using deep convolutional neural networks (CNNs) for damage detection. These networks are trained to recognize patterns and anomalies in structural data, improving the accuracy of damage assessments even under challenging conditions such as image noise.
Innovations in Structural Health Monitoring
Variational Mode Decomposition (VMD) and GARCH Model
One of Gharehbaghi’s significant contributions is developing a data-driven approach that combines VMD and the GARCH model for damage detection. This method decomposes response signals into intrinsic mode functions (IMFs) and uses the GARCH model to represent their statistics. The resulting feature vector is then processed using kernel-based principal component analysis (PCA) and linear discriminant analysis (LDA) to reduce redundancy and enhance feature informativeness.
Deep Convolutional Neural Networks (CNNs)
Gharehbaghi has also explored deep CNNs, using them to detect damage to some networks and perform feature extraction and classification in a single process, simplifying the damage detection workflow and improving its robustness against noisy data.
Case Studies and Practical Applications
Damage Detection in Real-World Structures
Gharehbaghi’s methodologies have been tested and validated on various real-world structures. For instance, his techniques have been applied to detect damages in bridges and high-rise buildings, demonstrating their effectiveness in diverse environments.
Collaborative Research
Gharehbaghi has collaborated with other experts in the field, such as Andy Nguyen and Seyedali Mirjalili, to refine and validate his techniques further. These collaborations have resulted in several high-impact publications contributing to the global knowledge base on structural health monitoring.
Impact and Future Directions
Enhancing Infrastructure Safety
Gharehbaghi’s work has significant practical implications. By improving the accuracy and reliability of damage detection methods, his research helps ensure the safety and longevity of critical infrastructure. This, in turn, reduces maintenance costs and prevents catastrophic failures.
Future Research Directions
Looking ahead, Gharehbaghi aims to integrate advanced machine learning techniques with real-time monitoring systems further. This integration will enable continuous structural health assessment and prompt interventions, enhancing the infrastructure’s overall resilience.
Conclusion
Vahid Reza Gharehbaghi’s contributions to structural health monitoring represent a significant advancement in civil engineering. His innovative use of machine learning and deep learning techniques has set a new standard for damage detection and assessment, ensuring the safety and sustainability of modern infrastructure. As his research continues to evolve, it promises to bring even more sophisticated solutions to the challenges of structural health monitoring, solidifying his position as a leading figure in this critical area of study.